Have you ever wondered where music or podcast recommendations come from? How do streaming services personalize your audio experience?
In short, they use machine learning (ML). And these ML algorithms are gaining mainstream adoption.
Machine learning statistics reflect the growing popularity of ML technologies in various industries. The global ML market is projected to grow to $209.91 billion by 2029.
Music streaming services like Spotify, Apple Music, SoundCloud, Google Play Music, and Tidal use personal data and machine learning to predict which tracks are most relevant to a particular audience.
Let’s see how ML engines can tailor service offerings to fit everyone’s tastes so well.
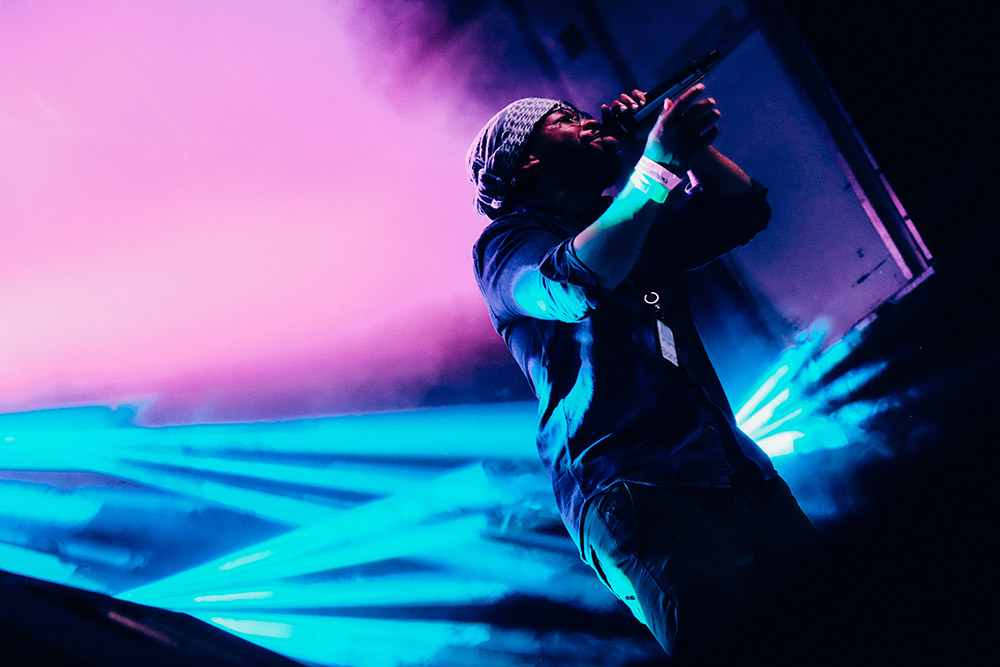
What is a music recommendation system (MRS)?
Sorting digital music causes information fatigue. Therefore, a music recommendation system has emerged to automatically personalize the experience by suggesting appropriate songs to users based on the characteristics of previously listened tracks.
Personalization creates relevant and engaging content for every user. It increases interaction with a product, site, service, or application. MRS uses ML algorithms and data, creating intelligent models that predict customer intent and provide highly customized recommendations.
A music recommendation solution can bring benefits to users and service providers. From a business standpoint, a successful music recommendation system strikes a balance between researching listener preferences and using data for recommendations. It boosts customer satisfaction and loyalty, helping providers keep customers on their platform for the long term. Users, in turn, get music and podcast content that perfectly fits their tastes.
Why streaming businesses use a music recommendation system (MRS)
Maximize user engagement
Music recommendation algorithms suggest personalized audio experiences like custom playlists, nearby concerts from their favorite artists or podcasters, and more. These recommendations keep listeners maximally engaged around similar styles and genres they like.
Increase conversion and loyalty
Recommendations help convert visitors into paying customers and keep them on the service as long as possible. Personalized music playlists encourage users to spend more time listening to their favorite tracks. It boosts customer satisfaction and loyalty.
Enable music discovery
MRS ensures high visibility of every track published on a platform. ML algorithms analyze consumed music, artists, podcasts, speakers, genres, and descriptions in multiple languages and then suggest podcasts and music based on personal tastes.
Support musicians and podcasters
Personalized recommendations can also benefit creators and increase their recognition as ML algorithms connect artists with audiences. For example, young fans of the rock genre can discover new tracks faster.
In much the same way that a MRS recommends relevant content on streaming platforms, the social media algorithms do the same with social content. Podcasters and musicians use services like Wavve to turn their podcasts and music into engaging, easy-to-consume video clips. The social ML can then recommend these clips to the users who will most benefit from them.
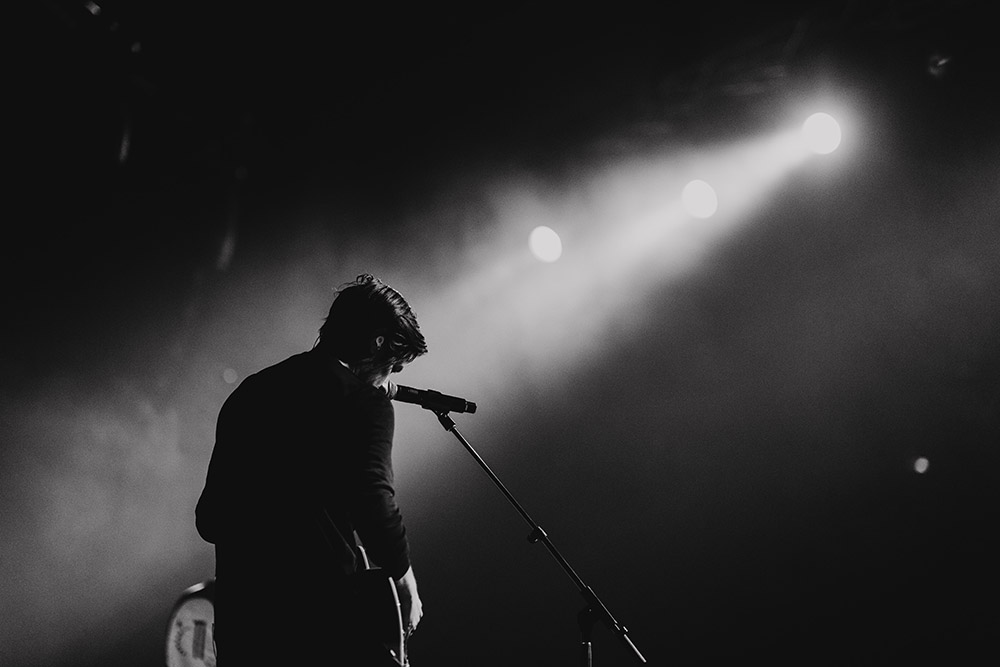
How do music streaming platforms know you so well?
Recommendation systems date back to the 1990s and use machine learning algorithms and personal data to predict preferences.
Music was one of the earliest examples of the use of recommendation systems. A listener’s preferences may vary depending on the mood, time, circumstances, or in response to different emotional needs.
ML-enabled systems can learn and adapt to a person’s taste to adjust to the contextual preferences of the listener during a listening session. Typically machine learning systems use techniques such as:
- Collaborative filtering (CF)
- Content-based filtering (CB)
- Hybrid of both methods
Content-based filtering
The content-based approach focuses on the similarity of specific items. This approach uses machine learning techniques like Naive Bayes classifiers and cluster analysis to determine which characteristics of an item to use to classify it.
When using a streaming music service, the listener likes or dislikes songs and creates playlists. The idea is to extract keywords from the song or podcast descriptions, compare them with the keywords of other tracks and further recommend similar ones to the user.
Pandora uses songs and artist properties to create a station that plays music with matching sonic properties. The platform relies on user feedback to refine station results, highlighting particular attributes when a user likes a track and other attributes when a user doesn’t like it.
Collaborative filtering
The collaborative recommendation filtering approach uses information about users’ overlapping behaviors or preferences, song ratings, and similarities to other listeners. Here, the most common machine learning method is the k-nearest neighbor’s (KNN) algorithm.
If two users express matching preferences, algorithms can recommend similar tunes. If the first user likes a particular song, the second user could also like that song, and vice versa. Such recommendation systems are considered more accurate due to their reliance on direct user interaction with the system.
Last.fm’s algorithm observes which playlists and tracks the user listens to regularly and compares them to the behavior of other users. The platform will then play songs from outside the user’s library that match users with similar tastes.
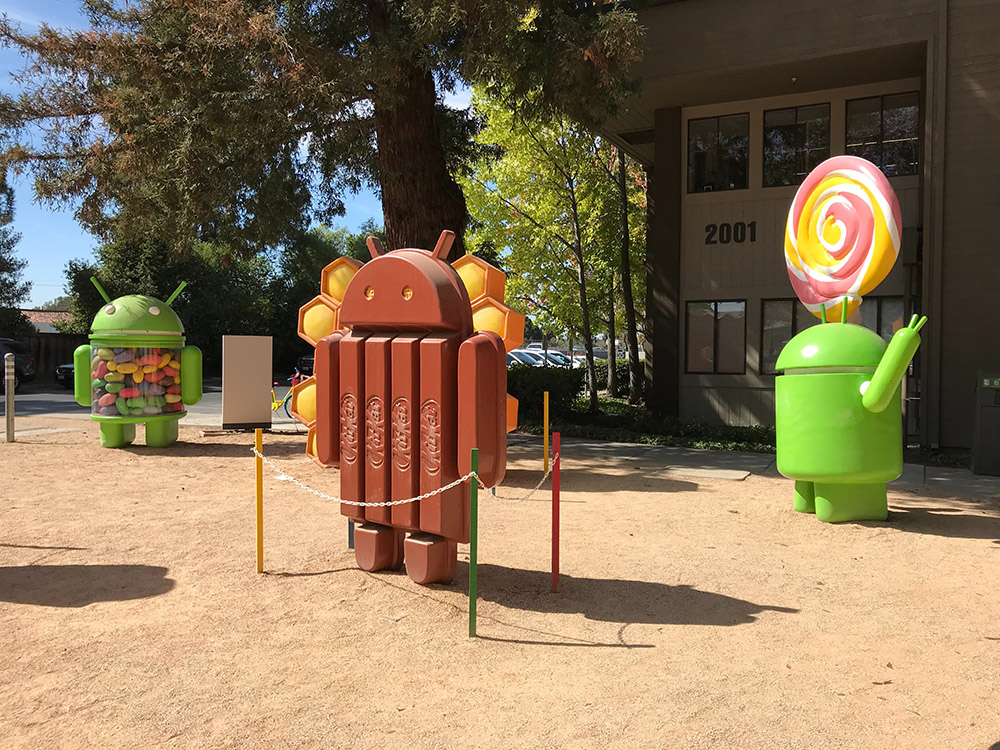
Google Play
With over 40 million songs, the Google Play Music library helps users find the right track on-demand with music personalization software.
Google uses machine learning technology in Google Play Music to select singles and albums released in the past two weeks based on listening history and general music preferences. The more a listener uses the platform, the better the playlist. The algorithm learns users’ favorite music and song preferences based on their previous behavior. For example, a workout playlist appears when you go to the gym. And if you are a fan of a particular artist, similar performers will appear in your playlist.
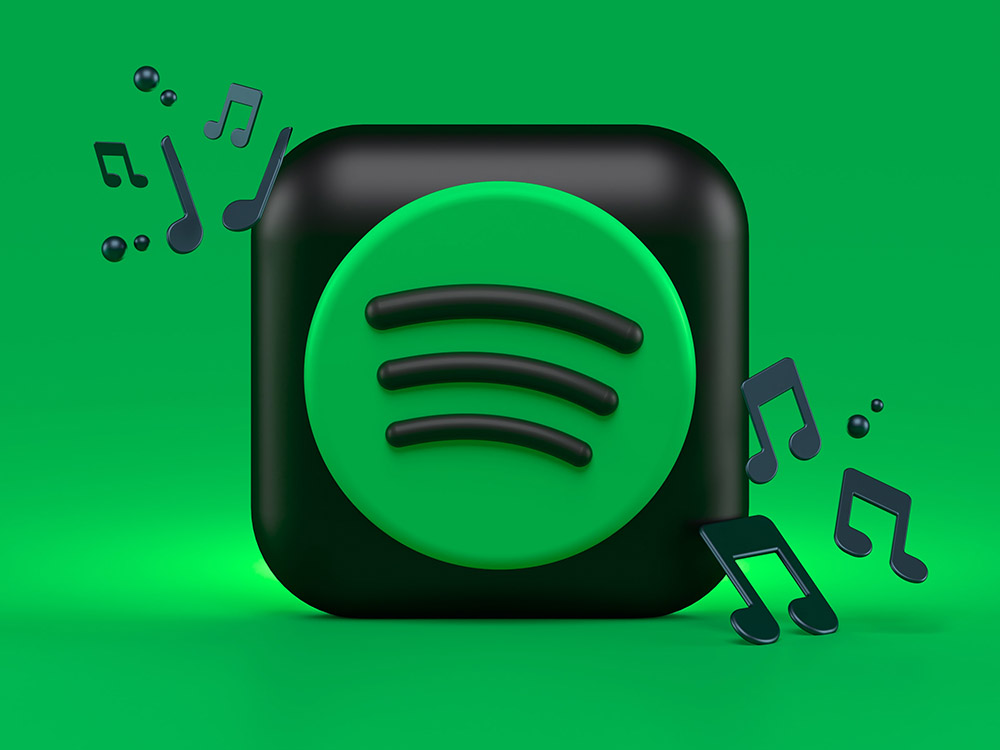
Spotify
Spotify sends a fresh new Discover Weekly playlist to 422 million users every Monday, consisting of songs that match each one’s taste profile.
Using a collaborative filtering approach, Spotify’s ML algorithms recognize patterns between playlists, songs, and users. The system analyzes song reviews to categorize music and learn from the platform’s user behavior.
ML draws parallels between your mood, feelings, and other people’s similar tastes. If someone else’s playlist contains at least a few of your favorite songs, Spotify will suggest tracks from that playlist to you. The algorithms then connect your preference profile and other users’ playlists and make a playlist that matches your taste.
In general, each user helps the algorithm improve by simply using the service:
- Adding songs you like to your playlist or library
- Skipping the songs you don’t like
- Listening to your favorites on repeat
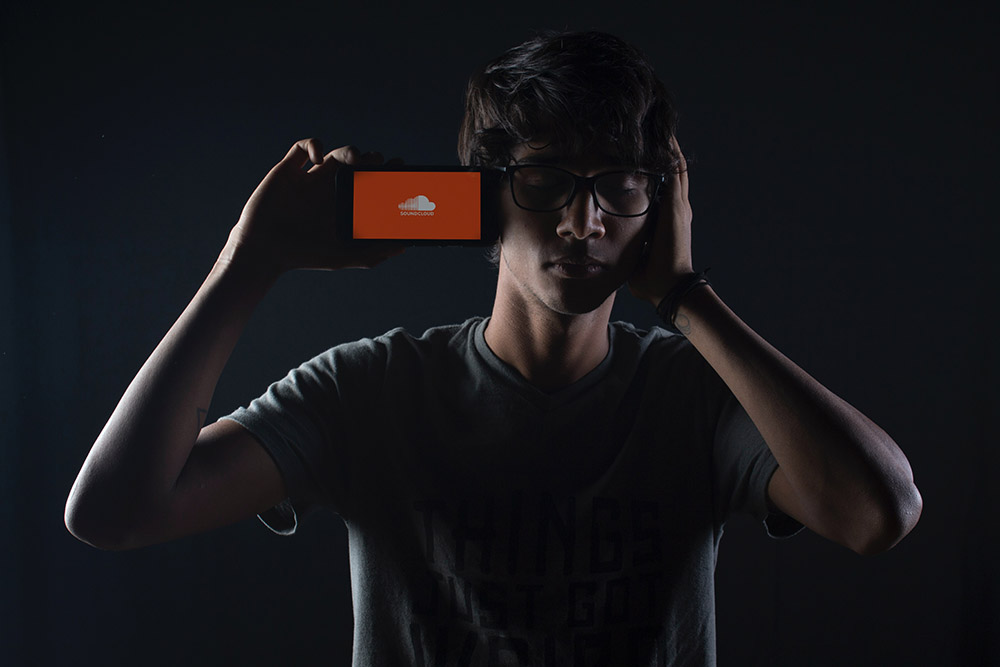
SoundCloud
SoundCloud is a streaming service where creators upload music and other recordings. The platform also has a recommendation system. The Upload feature uses ML to suggest new music uploaded to SoundCloud in the past few days based on tracks you’ve listened to earlier.
Generally speaking, Upload is an extension of another discovery feature called Suggested Tracks. The feature uses similar algorithms but dips into the platform’s broader catalog. While Suggested Tracks was like Discover Weekly, Upload is reminiscent of Spotify’s Release Radar, which also offers listeners new releases.
In addition to Upload and Suggested Tracks, SoundCloud’s other ML-based services include Who to Follow, Charts, and Stations.
The bottom line
Listeners rely on music platforms that provide an advanced ecosystem and feature set to manage their personal music library and discover new content based on individual preferences.
Machine learning has quickly become an essential technology, personalizing the user experience by delivering quality music and podcast content matching user preferences. So don’t be surprised if Spotify, Google, and Apple Music offer exactly the music and playlists you want to hear.
As creators, understanding these recommendation algorithms will help you improve discovery and build your audience.
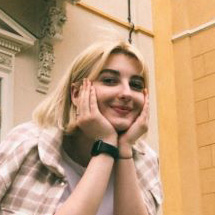
Alexandra Datsenko is a Technology Research Analyst at Itransition, a software development company headquartered in Denver. With a strong background in IT, she focuses on technologies driving innovation across industries and reshaping our daily lives.